A Bayesian Method of Forecast Averaging for Models Known only by Their Historic Outputs: An Application to the BCRA's REM
Working papers | 2006 | N 7
Keywords:
Bayesian method, Forecasting, REMAbstract
Similar to other Central Banks, the BCRA publishes monthly a REM that summarizes the projections of a group of economic analysts and consultants who volunteer to participate in the program. The BCRA publishes only the median and the standard deviation of the sample received. The logic for using these statistics is that all participants are to be treated equally. Under the assumption that some forecasters have better underlying models than others, one might be able to improve the accuracy of the aggregate forecast by giving greater priority to those who have historically predicted better. The BCRA does not have access to the models used to make the predictions, only the forecasts are provided. An averaging method that puts higher weights on predictions of those forecasters who have done best in the past should be able to produce a better aggregate forecast. The problem is how to determine these weights. In this paper, we develop a Bayesian averaging method what can do that well. The aggregate forecasts that come from our Bayesian averaging provide statistically better forecasts than the mean, median, best model, five best models and others method traditionally used. In particular, the method developed in this paper is much better at detecting changes in the trends of the variables. The aggregate predictions published from the REM provide information that is useful, not only for monetary and economic policy decisions, but also for the consumptions and business decisions of private economic agents. Improving these forecasts are of benefit to all members of the economy.
JEL classification: C53, E37
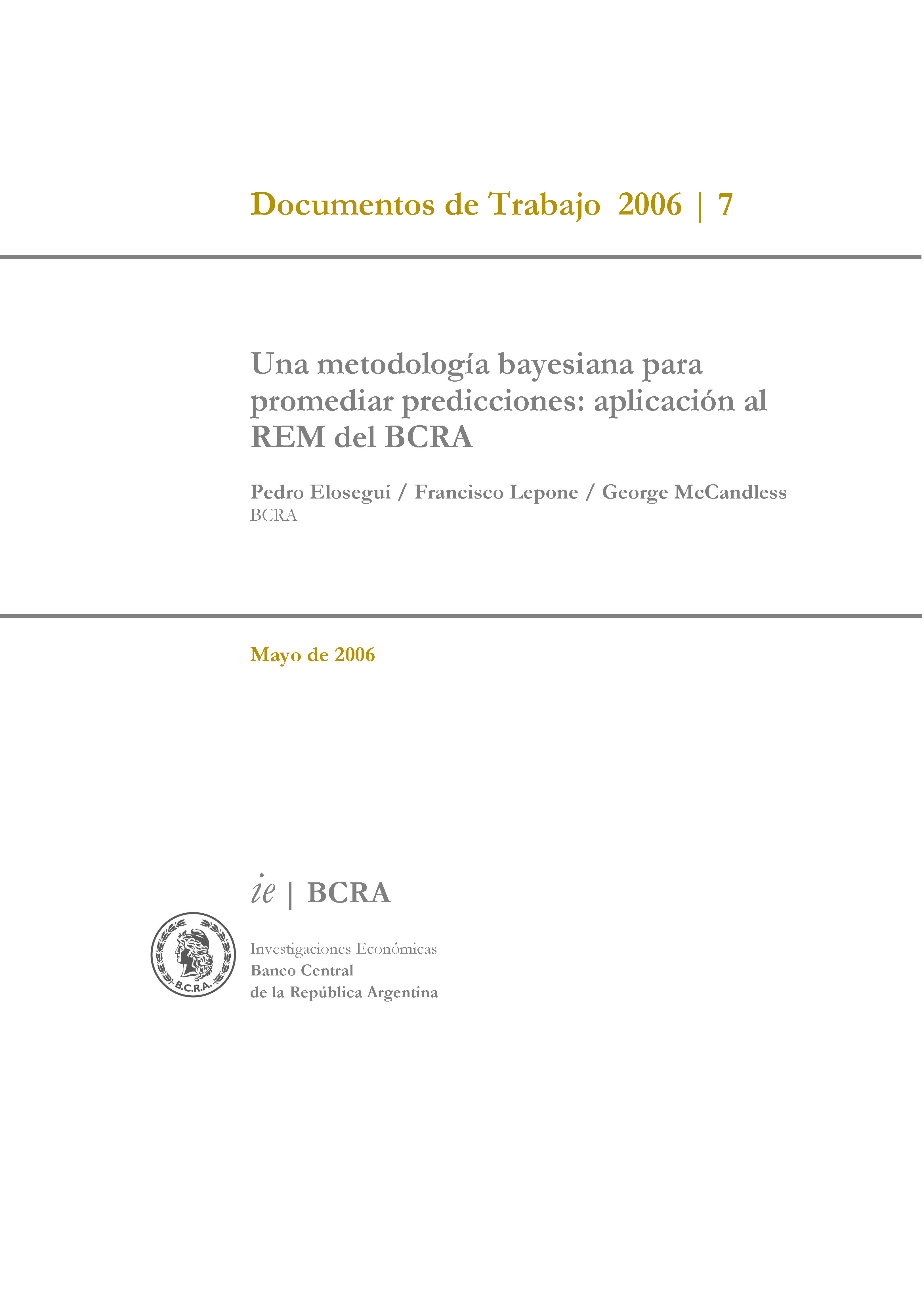